Research
Geometric deep learning
In Geometric Deep Learning one deals with data that has a non-Eucleadian structure, either by characterizing the structure of the data or by analyzing functions defined on a non-Euclidean domain. It is reasonable to require that a predictive model outputs the same results for two data points that are the same, independently of how the data is stored on a computer. Two different ways of storing a graph should not produce two different results, as two different charts on a manifold should not influence the content of the data on the manifold. Geometric deep learning deals with these issues. [1]
In my research I focus on learning vector fields and tensor fields on manifolds, with special attention to the group of 3D rotations \(SO(3)\), based on results by [2] and [3].
[1] M. M. Bronstein, J. Bruna, Y. LeCun, A. Szlam, and P. Vandergheynst. Geometric deep learning: going beyond euclidean data. IEEE Signal Processing Magazine, 34(4):18–42, 2017.
[2] Cohen, T. S., Geiger, M., Koehler, J., & Welling, M. (2018). Spherical CNNs. arXiv, 1801.10130.
[3] Esteves, C., Allen-Blanchette, C., Makadia, A., & Daniilidis, K. (2020). Learning SO(3) Equivariant Representations with Spherical CNNs. Int. J. Comput. Vision, 128(3), 588–600. doi: 10.1007/s11263-019-01220-1
Statistics on manifolds
In a general Riemannian manifold computing distances between two points is not an easy task. One could do that by following the geodesics and optimizing the initial velocity by gradient descent, but this is often a computationally intensive operation. What we are interested in studying is the possibility of defining a commuter metric on such manifolds by preprocessing the manifold and computing distances between a set of points of interest. [1]
[1] Grong, E., & Sommer, S. (2021). Most probable paths for anisotropic Brownian motions on manifolds. arXiv, 2110.15634
Neurogeometry of the visual cortex
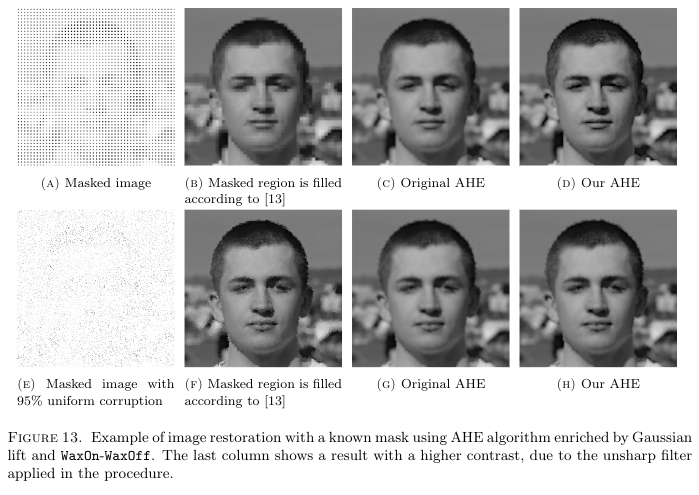
This project is based on previous work by Citti and Sarti [1] and subsequent works by Boscain et al. [2,3,4] to develop new procedures and algorithms for PDE-based image restoration.
Equipping the rototranslation group \(SE(2)\) with a sub-Riemannian structure inspired by the visual cortex V1, we propose algorithms for image inpainting and enhancement based on hypoelliptic diffusion. We innovate on previous implementations of the methods by Citti, Sarti and Boscain et al., by proposing an alternative that prevents fading and capable of producing sharper results in a procedure that we call WaxOn-WaxOff. We also exploit the sub-Riemannian structure to define a completely new unsharp using \(SE(2)\), analogous of the classical unsharp filter for 2D image processing, with applications to image enhancement. We demonstrate our method on blood vessels enhancement in retinal scans. [5]
[1] G Citti and A Sarti. “A cortical based model of perceptual completion in the Roto-translation space”. en. In: J. Math. Imaging Vis. 24.3 (May 2006), pp. 307– 326.
[2] Ugo Boscain et al. “Anthropomorphic Image Reconstruction via Hypoelliptic Diffusion”. In: SIAM j. control optim. 50.3 (Jan. 2012), pp. 1309–1336.
[3] U Boscain et al. “Hypoelliptic diffusion and human vision: A semidiscrete new twist”. en. In: SIAM J. Imaging Sci. 7.2 (Jan. 2014), pp. 669–695.
[4] Ugo V Boscain et al. “Highly corrupted image inpainting through hypoelliptic diffusion”. en. In: J. Math. Imaging Vis. 60.8 (Oct. 2018), pp. 1231–1245.
[5]Ballerin, F., & Grong, E. (2023). Geometry of the Visual Cortex with Applications to Image Inpainting and Enhancement. arXiv, 2308.07652.